Artificial Intelligence aims to build computer programs that can undertake tasks that would traditionally require human intelligence to complete. In the healthcare space, AI is seen as a helping hand to clinicians. As per estimates global software revenue from its top cases in healthcare will grow from $3.7 billion in 2019 to nearly $67 billion by 2027. Over the past few years, the AI innovation in healthcare has been overwhelming, more than in other AI-disrupted industries. It is due this fact, healthtech startups worldwide are already jumping at the opportunity, especially with the digital needs of the post-pandemic world in mind.
Current state-of-the-art AI algorithms require immense amounts of data to learn, in most cases, the more data the better. Hospitals and research institutions need to combine their data reserves if they want a pool of data that is large and diverse enough to be useful. But the idea of centralizing reams of sensitive medical information in the hands of tech companies has repeatedly and unsurprisingly proved intensely unpopular. As a result, research on diagnostic uses of AI has stayed narrow in scope and applicability. You can’t deploy a breast cancer detection model around the world when it’s only been trained on a few thousand patients from the same hospital.
All this could change with federated learning. The technique can train a model using data stored at multiple different hospitals without that data ever leaving a hospital’s premises or touching a tech company’s servers. By implementing this type of system medical research organizations can successfully fulfil AI model training challenges, diversify revenue streams & foster collaborative, and enable data-driven innovation.
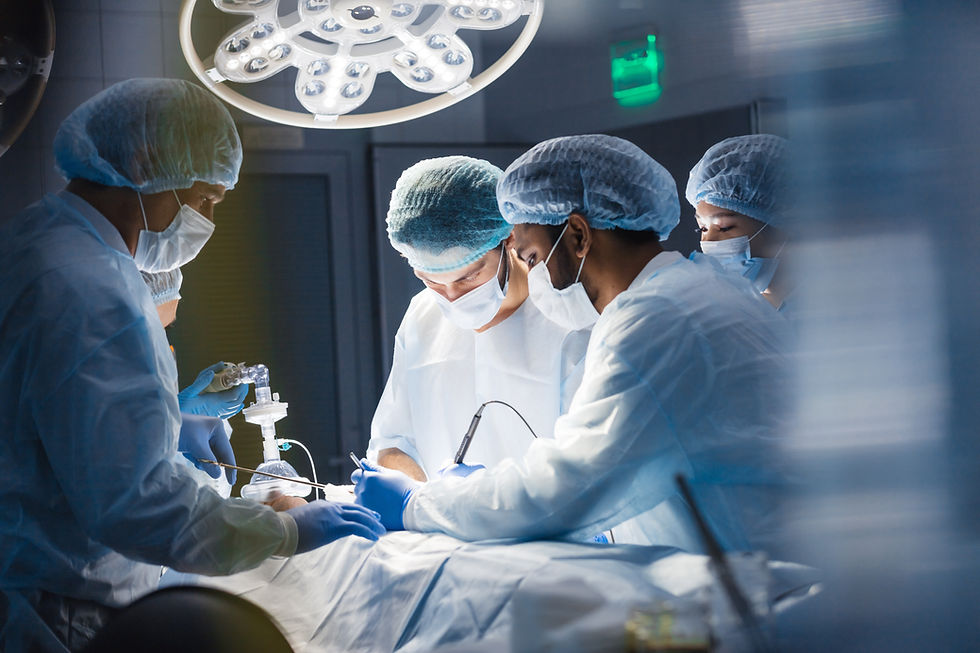
Comments